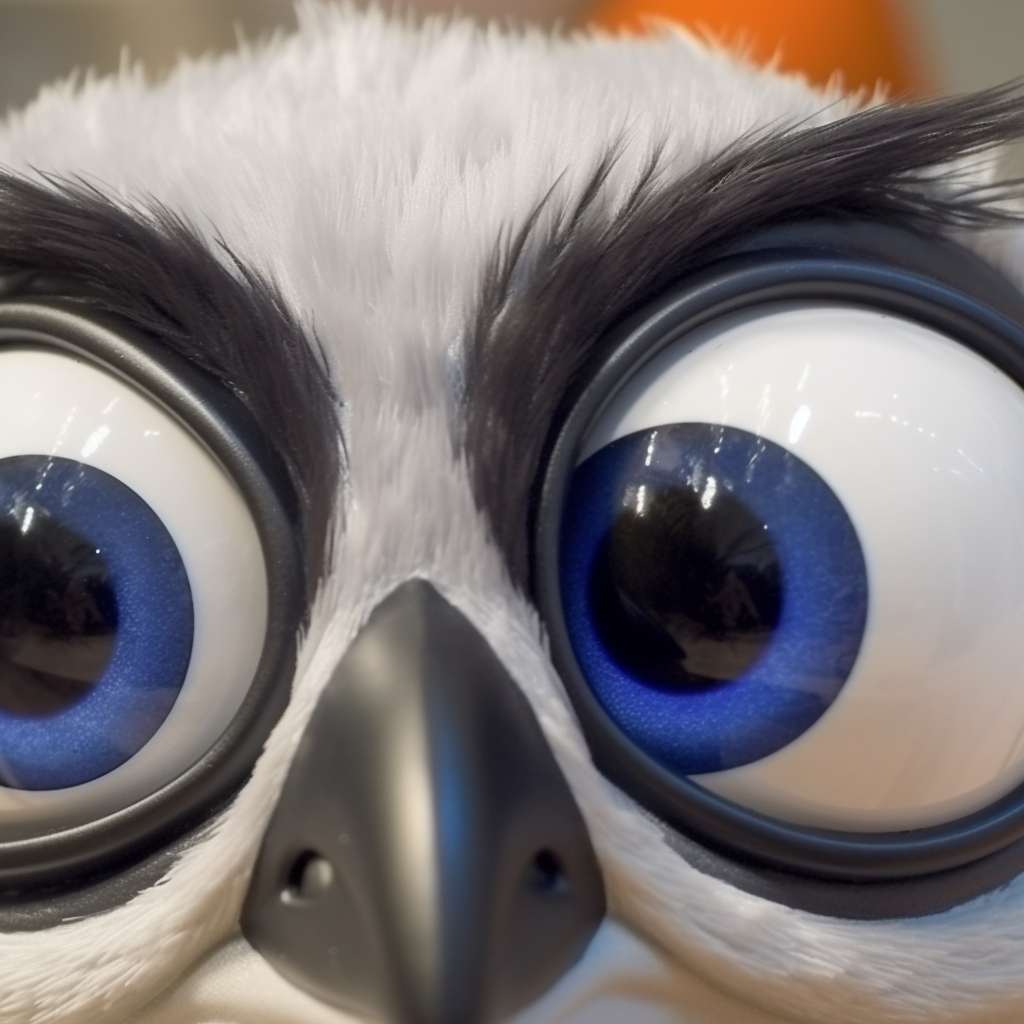
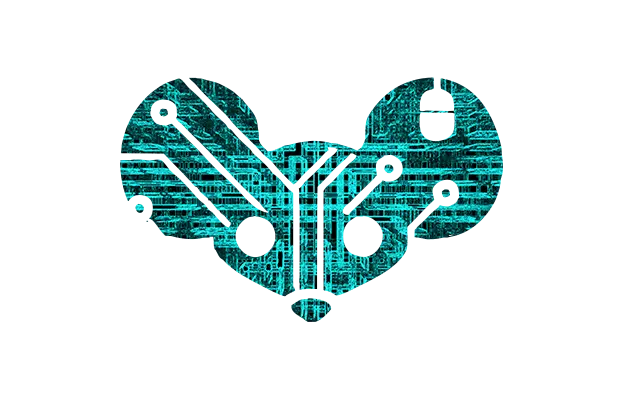
Hot take: what most people call AI (large language and diffusion models) is, in fact, part of peak capitalism:
- relies on ill gotten gains (training data obtained without permission, payment or licensing)
- aims to remove human workers from the workforce within a system that (for many) requires them to work because capitalism has removed the bulk of social safety netting
- currently has no real route to profit at any reasonable price point
- speculative at best
- reinforces the concentration of power amongst a few tech firms
- will likely also result in regulatory capture with the large firms getting legislation passed that only they can provide “AI” safely
I could go on but hopefully that’s adequate as a PoV.
“AI” is just one of cherries on top of late stage capitalism that embodies the worst of all it.
So I don’t disagree - but felt compelled to share.
Won’t you be my Neighbor is a wonderful documentary whether you’re a fan or not.