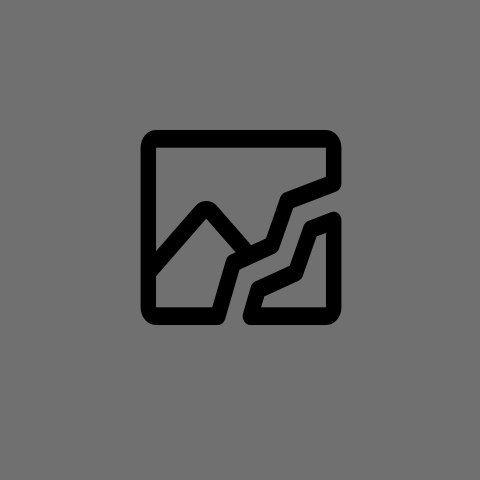
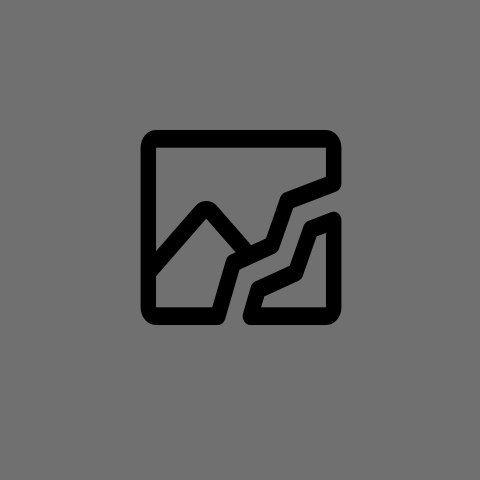
Regular users can use Gemini, Deepseek, Meta AI, and there will probably be many more services in the future.
Regular users can use Gemini, Deepseek, Meta AI, and there will probably be many more services in the future.
NFS gives me the best performance. I’ve tried GlusterFS (not at home, for work), and it was kind of a pain to set up and maintain.
If it works, I don’t update unless I’m bored or something. I also spread things out on multiple machines, so there’s less chance of stuff happening like you describe with the charts feature going away. My NAS is pretty much just a NAS now.
You can probably backup your configs/data, upgrade, then deploy jellyfin again, restore, and reconfigure. You should probably backup your data on your ZFS pool. But, I recently updated to the latest TrueNas Scale from ~5 year old FreeBSD version of TrueNas and the pools still worked fine (none of the “apps” or jails worked, obviously). The upgrade process even ported my service configurations over. I didn’t care about much of the data in the pools, so only backed up the most important stuff.
I personally use a dual core pentium with 16GB of RAM. When I first installed TrueNas (FreeNas back then), I only had 8GB of RAM, but that proved to be not enough to run all the services I wanted, so I would suggest 12-16GB. Depending on the services you want to run any multi-core x86 CPU that allows 16GB of RAM to be used should be adequate. I believe TrueNas recommends ECC RAM, but I don’t think using consumer grade RAM and hardware has caused me any problems. I’m also using an old SSD for the system drive, which I is recommended now (I used to use 2 mirrored USB thumb drives, buy that’s not recommended anymore). Very importantly, make sure the HDD(s) you get are not shingled drives; made that mistake initially, and performance was ridiculously bad.
Yeah, I was disappointment when I bought a very expensive Galaxy S22 to replace my old Moto G whose charging port wore out,. The S22 had worse battery life, camera, and no noticeable performance improvements. Recently, my S22 stopped charging, and I just bought a “Mint”-grade used Pixel 6 and installed GrapheneOS on it. Happy so far, and it’s nice to be able to block network access to all apps, including Google’s.
Some of the “open” models seem to have augmented their training data with OpenAI and Anthropic requests (I. E. they sometimes say they’re ChatGPT or Claude). I guess that may be considered piracy. There are a lot of customer service bots that just hook into OpenAI APIs and don’t have a lot of guardrails, so you can do stuff like ask a car dealership’s customer service to write you Python code. Actual piracy would require someone leaking the model.
I’m curious if ByteDance could just create a new legal entity and call it TikTak or something.
If you have to verify children’s identity, you have to verify everyone’s identity. This is part of KOSA. https://www.eff.org/deeplinks/2024/12/kids-online-safety-act-continues-threaten-our-rights-online-year-review-2024
Oldest I got is limited to 16GB (excluding rPis). My main desktop is limited to 32GB which is annoying, because I sometimes need more. But, I have a home server with 128GB of RAM that I can use when it’s not doing other stuff. I once needed more than 128GB of RAM (to run optimizations on a large ONNX model, iirc), so had to spin up an EC2 instance with 512GB of RAM.
I just use Joplin, encrypted, and synced through dropbox. Tried logseq, but never really figured out how to use its features effectively. The notebook/note model of Joplin seems more natural to me. My coding/scripting stuff mostly just goes into git repos.
The PC I’m using as a little NAS usually draws around 75 watt. My jellyfin and general home server draws about 50 watt while idle but can jump up to 150 watt. Most of the components are very old. I know I could get the power usage down significantly by using newer components, but not sure if the electricity use outweighs the cost of sending them to the landfill and creating demand for more newer components to be manufactured.
CK2 - 400h
Fallout NV (guessing most of this has been TTW) - 190h
Stellaris - 180h
Xcom 2 - 140h
GTA 5 - 99h
Cities Skylines - 95h
Skyrim - 90h
Civ5 - 85h
Xcom - 83h
The other games I’ve played are pretty much the standard play-through times. (< 70h)
I’m loading up on vacuum tubes.
Last time I looked it up and calculated it, these large models are trained on something like only 7x the tokens as the number of parameters they have. If you thought of it like compression, a 1:7 ratio for lossless text compression is perfectly possible.
I think the models can still output a lot of stuff verbatim if you try to get them to, you just hit the guardrails they put in place. Seems to work fine for public domain stuff. E.g. “Give me the first 50 lines from Romeo and Juliette.” (albeit with a TOS warning, lol). “Give me the first few paragraphs of Dune.” seems to hit a guardrail, or maybe just forced through reinforcement learning.
A preprint paper was released recently that detailed how to get around RL by controlling the first few tokens of a model’s output, showing the “unsafe” data is still in there.
I think TikTok appeased the right by changing their algorithm. Charlie Kirk is apparently doing extremely well on the platform now.
You can also just install the libreelec os (Kodi), and install the Jellyfin Kodi addon. Haven’t tried that addon. I used to use Kodi when I had only one TV, and liked it. Now that I have 2 Android TVs, just installing Jellyfin on the TVs works fine. I might go back to rPIs and disconnect my TVs from the internet though.
I use GPT (4o, premium) a lot, and yes, I still sometimes experience source hallucinations. It also will sometimes hallucinate incorrect things not in the source. I get better results when I tell it not to browse. The large context of processing web pages seems to hurt its “performance.” I would never trust gen AI for a recipe. I usually just use Kagi to search for recipes and have it set to promote results from recipe sites I like.
Hmm. I just assumed 14B was distilled from 72B, because that’s what I thought llama was doing, and that would just make sense. On further research it’s not clear if llama did the traditional teacher method or just trained the smaller models on synthetic data generated from a large model. I suppose training smaller models on a larger amount of data generated by larger models is similar though. It does seem like Qwen was also trained on synthetic data, because it sometimes thinks it’s Claude, lol.
Thanks for the tip on Medius. Just tried it out, and it does seem better than Qwen 14B.
Larger models train faster (need less compute), for reasons not fully understood. These large models can then be used as teachers to train smaller models more efficiently. I’ve used Qwen 14B (14 billion parameters, quantized to 6-bit integers), and it’s not too much worse than these very large models.
Lately, I’ve been thinking of LLMs as lossy text/idea compression with content-addressable memory. And 10.5GB is pretty good compression for all the “knowledge” they seem to retain.
Oh, I forgot about Claude. Last time I tried it, it seemed on par or even better that ChatGPT-4o (but was missing features like browsing).